Research
The Kuijjer group's research program aims at understanding the molecular mechanisms that drive cancer development, progression, and heterogeneity. Our driving hypothesis is that the complex clinical phenotypes we observe in cancer cannot be adequately defined by individual layers of molecular data. Instead, we must consider the underlying network of interactions between the different biological components that can drive cancer phenotypes. To do so, we develop computational approaches that place genomic data into the context of large-scale, genome-wide regulatory networks.
- We specifically focus on three Research Lines (RL, see Fig 1): RL1. development of computational tools that leverage approaches from network science and artificial intelligence to map gene regulatory landscapes for individual cancer patients and single cells; RL2. development of approaches to statistically compare large-scale networks and to integrate these networks with other data types such as omics data and clinical features; and RL3. the development of approaches to model regulatory networks for newly emerging data types, such as spatial omics data. Through applications of our network models to cancer data, we aim to study how network rewiring may drive cancer development, progression, and clinical phenotypes
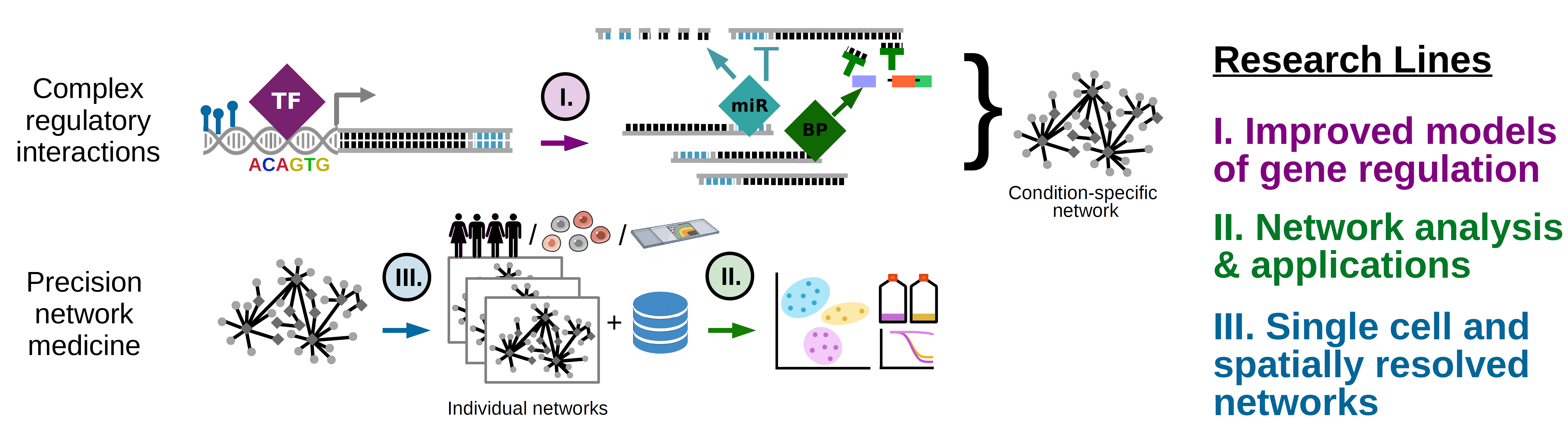
- The ultimate goal of our research program is to use computational modeling to shed light on the interplay between various regulatory mechanisms that drive cancer. Supported by experimental validations from our collaborators, we hope that, in the long term, this approach has the potential to contribute to improving cancer diagnosis, treatment, and outcomes.
RL1. Fine-tuned modeling of gene regulatory interactions
- Our group has been on the forefront of network modeling of gene regulatory mechanisms, for example to gain understanding on tissue-specificity of gene expression (Sonawane et al.). We specifically work with and develop approaches use domain knowledge, for example on TF and miRNA binding, to integrate multi-modal data. This includes methods that are based on message passing (Fig 2, e.g. with PyPanda and PUMA), which we have mostly used to model regulatory mechanisms, such as predicting transcription factor interactions in promoter regions of their target genes. In addition, we design approaches that allow for more precise network modeling (e.g. SNAIL). More recently, we have expanded our models of gene regulation by developing deep learning approaches that leverage domain knowledge on data dependencies (e.g. between chromatin states and gene expression) to integrate multi-modal single-cell data (e.g. CAVACHON).
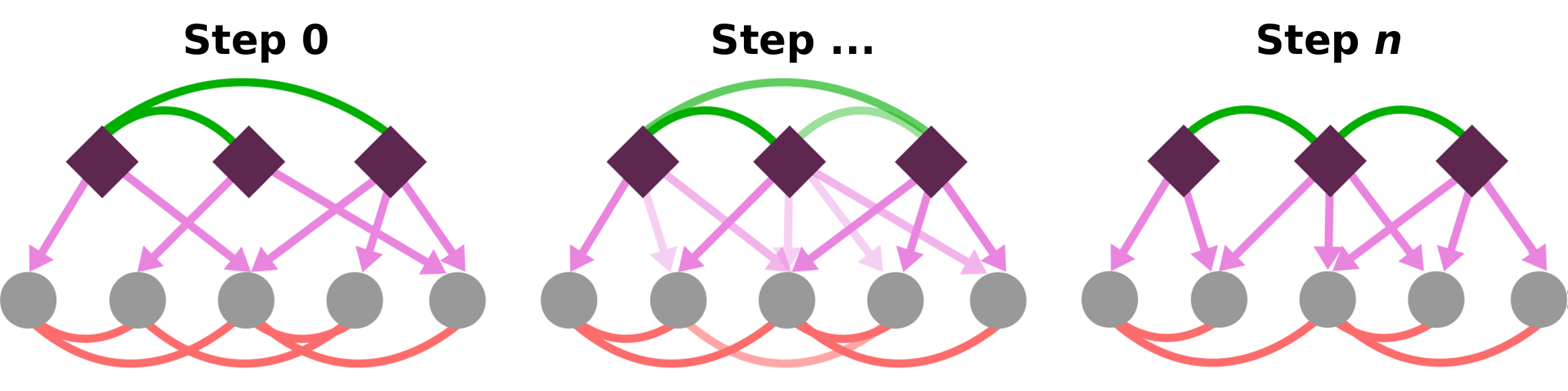
RL2. Integration and analysis of regulatory networks with multi-modal data
- Network reconstruction algorithms often draw on large numbers of measured expression samples to tease out subtle signals and infer connections between genes or gene products. The result is an aggregate network model representing a single estimate for edge likelihoods. While informative, aggregate models fail to capture the heterogeneity represented in a population. Our group has been a pioneer in single-sample network modeling (LIONESS, lionessR, DeMarzio et al.), which has opened up the possibility to analyze networks in the context of clinical features (e.g. Lopes-Ramos et al.). Expanding our single-sample network modeling toolbox, we developed approaches for fine-tuned network analysis to characterize tumor heterogeneity (PORCUPINE). We currently focus on approaches integrate and analyze regulatory networks with multi-modal data, as well as new statistical approaches for to study tumor evolution in the context of gene regulatory network rewiring. These new approaches can, for example, help identify novel regulatory subtypes in cancer for treatment stratification, identify specific regulatory interactions that drive cancer phenotypes and progression, and potential new targets for treatment of disrupted gene regulation.
RL3. A high resolution map of regulatory interactions in cancer
- Finally, we focus on developing new tools for fine-tuned modeling and analysis of single-cell regulatory networks (e.g. SCORPION) and spatially resolved networks and deep learning based methods that integrate multi-modal single-cell data to uncover potential new cell subpopulations. We aim to integrate single-cell and spatial regulatory information with drug response profiles to predict candidate drugs for specific (e.g. therapy-resistant) cell subpopulations (based on our tool retriever). This may uncover heterogeneity of regulatory programs in cancer cells and the tumor microenvironment, as well as potential new targets for treatment that specifically target aggressive cancer cell subpopulations.